What is TSS loading data?
TSS (Time Series Signal) loading data refers to the process of importing time-stamped data into a system or database for further analysis and processing. It involves converting raw data into a structured format that can be efficiently processed and utilized for various purposes, such as predictive analytics, anomaly detection, and forecasting.
TSS loading data plays a crucial role in many industries and applications, including finance, healthcare, manufacturing, and energy. It enables organizations to leverage historical and real-time data to gain insights into trends, patterns, and correlations, ultimately leading to better decision-making and improved outcomes.
TSS Loading Data
TSS loading data, a crucial aspect of data management and analysis, involves importing time-stamped data into a system or database for further processing. This process plays a vital role in various industries and applications.
- Data Import: Acquiring data from various sources.
- Time Series: Data with timestamps, capturing temporal patterns.
- Structured Format: Converting raw data into a consistent structure.
- Analysis: Uncovering insights from time-stamped data.
- Predictive Analytics: Forecasting future trends based on historical data.
- Anomaly Detection: Identifying unusual patterns or deviations.
- Decision-Making: Informing decisions based on data-driven insights.
- Optimization: Improving processes and outcomes using data analysis.
The key aspects of TSS loading data are interconnected and essential for effective data management and analysis. By understanding and utilizing these aspects, organizations can gain valuable insights from their time-stamped data, leading to improved decision-making, enhanced operational efficiency, and better outcomes.
1. Data Import
Data import, a fundamental aspect of TSS loading data, involves acquiring data from diverse sources to create a comprehensive dataset for analysis. This process is crucial as it determines the quality and scope of insights that can be derived from the data.
When importing data, it is essential to consider the following factors:
- Data Source Reliability: Ensuring the accuracy and credibility of data sources is critical to avoid biased or incorrect analysis.
- Data Format Compatibility: Data from different sources may have varying formats, so it must be converted into a consistent structure for seamless integration and analysis.
- Data Cleaning and Preprocessing: Removing duplicate or erroneous data, as well as handling missing values, is crucial to ensure data integrity.
By carefully managing the data import process, organizations can create a robust and reliable dataset that forms the foundation for accurate and insightful TSS analysis.
2. Time Series
Time series data, characterized by its timestamps, is a fundamental component of TSS loading data. Timestamps provide a chronological context, allowing for the analysis of data over time and the identification of trends, patterns, and correlations.
The significance of time series data lies in its ability to capture the temporal dynamics of a system or process. By understanding how data changes over time, organizations can gain valuable insights into the underlying mechanisms and relationships.
For instance, in financial markets, time series data of stock prices enables analysts to track price movements, identify trading patterns, and make informed investment decisions. Similarly, in healthcare, time series data of patient vital signs can help medical professionals monitor patient health, detect anomalies, and provide timely interventions.
Overall, the incorporation of time series data into TSS loading data provides a powerful tool for organizations to analyze and understand the temporal evolution of systems and processes, leading to improved decision-making and enhanced outcomes.
3. Structured Format
In the context of TSS loading data, structured format plays a pivotal role in ensuring data integrity and enabling efficient analysis. It involves converting raw data, which can be unstructured and heterogeneous, into a consistent and organized structure that facilitates seamless processing and interpretation.
- Data Standardization: Raw data often comes in varying formats, making it challenging to analyze. Structured format standardizes data into a common format, ensuring consistency and compatibility.
- Data Cleaning and Transformation: Data cleaning involves removing duplicate or erroneous data, while data transformation involves converting data into a format suitable for analysis. Structured format streamlines these processes.
- Data Integration: When data is collected from multiple sources, it is essential to integrate it into a single, cohesive structure. Structured format enables seamless integration, allowing for comprehensive analysis.
- Enhanced Analysis: A structured format makes data more manageable and accessible for analysis. It allows for the application of statistical techniques, machine learning algorithms, and other analytical methods to extract meaningful insights.
By converting raw data into a structured format, TSS loading data lays the foundation for accurate and reliable analysis. It ensures data integrity, facilitates efficient processing, and enables organizations to uncover valuable insights from their time-stamped data.
4. Analysis
Analysis plays a vital role in TSS (Time Series Signal) loading data, as it enables organizations to uncover valuable insights and patterns from their time-stamped data. This data can provide a wealth of information about system performance, customer behavior, and market trends, enabling organizations to make informed decisions and improve outcomes.
- Trend Analysis: By analyzing time-stamped data over time, organizations can identify trends and patterns that would otherwise remain hidden. This information can be used to forecast future events, optimize processes, and anticipate market changes.
- Anomaly Detection: Time-stamped data analysis can detect anomalies or deviations from expected patterns, indicating potential issues or opportunities. This information can be critical for risk management, fraud detection, and quality control.
- Correlation Analysis: By analyzing the relationships between different time series, organizations can identify correlations and dependencies that can inform decision-making. This information can be used to identify root causes, optimize resource allocation, and improve coordination.
- Predictive Modeling: Using machine learning algorithms, organizations can build predictive models based on their time-stamped data. These models can be used to forecast future outcomes, identify potential risks, and optimize decision-making.
In conclusion, the analysis of time-stamped data is a crucial aspect of TSS loading data, as it enables organizations to uncover valuable insights, identify patterns, and make informed decisions. By leveraging the power of time-stamped data, organizations can gain a competitive edge and achieve better outcomes.
5. Predictive Analytics
Predictive analytics, a powerful technique in TSS (Time Series Signal) loading data, leverages historical data to forecast future trends and patterns. By analyzing time-stamped data, organizations can gain insights into the underlying relationships and dynamics of their systems and processes.
- Trend Forecasting: Predictive analytics enables organizations to identify trends and patterns in time-stamped data. This information can be used to forecast future demand, predict market behavior, and anticipate changes in customer preferences.
- Risk Assessment: Predictive analytics can assess risks and identify potential threats by analyzing historical data. This information can be used to develop proactive strategies, mitigate risks, and ensure business continuity.
- Optimization: Predictive analytics can optimize processes and decision-making by identifying areas for improvement. This information can be used to streamline operations, reduce costs, and enhance efficiency.
- Personalized Experiences: Predictive analytics can be used to create personalized experiences for customers by analyzing their historical behavior. This information can be used to provide tailored recommendations, targeted marketing, and customized services.
In conclusion, predictive analytics plays a crucial role in TSS loading data by providing organizations with the ability to forecast future trends, assess risks, optimize processes, and create personalized experiences. By leveraging the power of historical data, organizations can gain a competitive edge and make informed decisions to improve their overall performance and outcomes.
6. Anomaly Detection
Anomaly detection plays a crucial role in TSS (Time Series Signal) loading data, as it enables organizations to identify unusual patterns or deviations from expected behavior. By analyzing time-stamped data, organizations can detect anomalies that may indicate potential issues, fraudulent activities, or opportunities for improvement.
The importance of anomaly detection in TSS loading data lies in its ability to provide early warnings and proactive insights. For example, in financial markets, anomaly detection can identify unusual trading patterns that may indicate market manipulation or insider trading. In healthcare, anomaly detection can help identify patients with rare diseases or predict the risk of complications based on their medical history.
To effectively implement anomaly detection in TSS loading data, organizations must consider factors such as data quality, noise reduction, and the selection of appropriate algorithms. By leveraging machine learning and statistical techniques, organizations can develop anomaly detection models that are tailored to their specific needs and data characteristics.
In conclusion, anomaly detection is an essential component of TSS loading data, as it enables organizations to identify unusual patterns and deviations that may have significant implications. By leveraging anomaly detection techniques, organizations can gain a deeper understanding of their systems and processes, mitigate risks, and make informed decisions to improve their overall performance and outcomes.
7. Decision-Making
In today's data-driven world, organizations rely heavily on data to make informed decisions and gain a competitive edge. TSS (Time Series Signal) loading data plays a crucial role in this process by providing a structured and organized foundation for analyzing time-stamped data.
- Data-Driven Insights: TSS loading data enables organizations to extract valuable insights from their time-stamped data. These insights can reveal trends, patterns, and anomalies that would otherwise be difficult to identify manually.
- Improved Accuracy: By leveraging data-driven insights, organizations can make more accurate and informed decisions. Data-driven insights provide a deeper understanding of the factors influencing performance, enabling organizations to make decisions based on evidence rather than guesswork.
- Enhanced Agility: TSS loading data empowers organizations to respond quickly to changing market conditions and customer demands. By analyzing time-stamped data, organizations can identify emerging trends and patterns, allowing them to adapt their strategies and make timely decisions.
- Reduced Risks: Data-driven insights can help organizations identify and mitigate risks. By analyzing historical data, organizations can identify potential risks and develop strategies to minimize their impact.
In conclusion, TSS loading data is essential for organizations seeking to make informed decisions based on data-driven insights. By providing a structured and organized foundation for analyzing time-stamped data, TSS loading data empowers organizations to gain a deeper understanding of their systems, processes, and customers. This, in turn, leads to improved accuracy, enhanced agility, reduced risks, and ultimately, better decision-making.
8. Optimization
In the realm of data management and analysis, TSS (Time Series Signal) loading data plays a pivotal role in optimizing processes and improving outcomes. By providing a structured and organized foundation for analyzing time-stamped data, TSS loading data empowers organizations to gain deeper insights into their systems, processes, and customers.
- Process Efficiency: TSS loading data enables organizations to identify bottlenecks and inefficiencies in their processes. By analyzing time-stamped data, organizations can pinpoint areas for improvement and implement changes to streamline operations and enhance efficiency.
- Resource Allocation: TSS loading data provides valuable insights into resource utilization. Organizations can analyze time-stamped data to determine how resources are being allocated and identify areas where optimization can lead to cost savings and improved performance.
- Customer Satisfaction: By analyzing time-stamped data related to customer interactions, organizations can gain a deeper understanding of customer behavior and preferences. This information can be used to improve customer satisfaction, enhance service delivery, and increase customer loyalty.
- Predictive Maintenance: TSS loading data is crucial for predictive maintenance strategies. By analyzing time-stamped data from sensors and equipment, organizations can identify potential issues and schedule maintenance before they escalate into major problems, reducing downtime and associated costs.
In conclusion, TSS loading data is a powerful tool for optimization, enabling organizations to improve processes, enhance outcomes, and gain a competitive edge. By leveraging time-stamped data, organizations can make data-driven decisions, identify areas for improvement, and ultimately achieve better performance and success.
FAQs on TSS Loading Data
This section addresses frequently asked questions and misconceptions surrounding TSS (Time Series Signal) loading data to provide a comprehensive understanding of its significance and applications.
Question 1: What is the importance of structuring time-stamped data for analysis?
Structuring time-stamped data into a consistent format is essential for efficient analysis. It ensures data integrity, enables seamless integration of data from multiple sources, and facilitates the application of analytical techniques.
Question 2: How does TSS loading data contribute to predictive analytics?
TSS loading data provides the foundation for predictive analytics by organizing time-stamped data in a manner that allows for the identification of patterns and trends. This data can be leveraged to build predictive models that forecast future outcomes and support informed decision-making.
Question 3: What are the benefits of anomaly detection in TSS loading data?
Anomaly detection in TSS loading data enables the identification of unusual patterns and deviations from expected behavior. This information is crucial for risk management, fraud prevention, and quality control, allowing organizations to take proactive measures and mitigate potential issues.
Question 4: How does TSS loading data support data-driven decision-making?
TSS loading data provides a structured and organized foundation for analyzing time-stamped data, enabling organizations to extract valuable insights and make informed decisions. Data-driven insights derived from TSS loading data enhance accuracy, agility, and risk mitigation, leading to better outcomes.
Question 5: What is the role of TSS loading data in process optimization?
TSS loading data plays a vital role in process optimization by providing insights into process efficiency, resource utilization, and customer behavior. Analyzing time-stamped data enables organizations to pinpoint bottlenecks, identify areas for improvement, and make data-driven decisions to enhance performance and outcomes.
In summary, TSS loading data is a fundamental aspect of data management and analysis, providing the foundation for predictive analytics, anomaly detection, data-driven decision-making, and process optimization. It empowers organizations to gain deeper insights, make informed choices, and achieve better outcomes.
Now that we have explored the importance and applications of TSS loading data, let's delve into the key steps involved in the TSS loading data process.
Conclusion
In conclusion, TSS (Time Series Signal) loading data is a critical process that underpins the analysis and utilization of time-stamped data. It provides a structured and organized foundation for extracting valuable insights, identifying patterns and trends, and making informed decisions.
Throughout this article, we have explored the importance of TSS loading data in various domains, including predictive analytics, anomaly detection, data-driven decision-making, and process optimization. By leveraging time-stamped data, organizations can gain a deeper understanding of their systems, processes, and customers, enabling them to improve performance, mitigate risks, and achieve better outcomes.
As the volume and complexity of time-stamped data continue to grow, TSS loading data will become increasingly essential for organizations seeking to harness the power of data for competitive advantage. By investing in robust TSS loading data strategies and technologies, organizations can unlock the full potential of their time-stamped data and drive innovation, growth, and success.
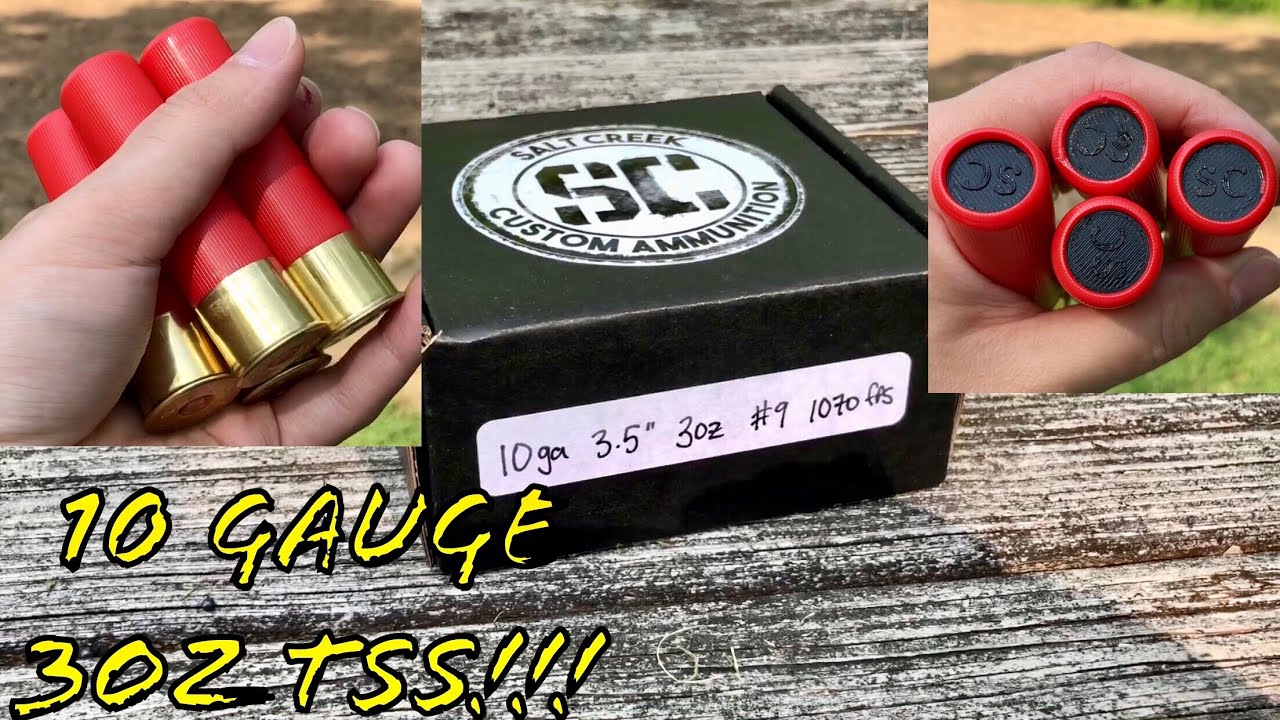

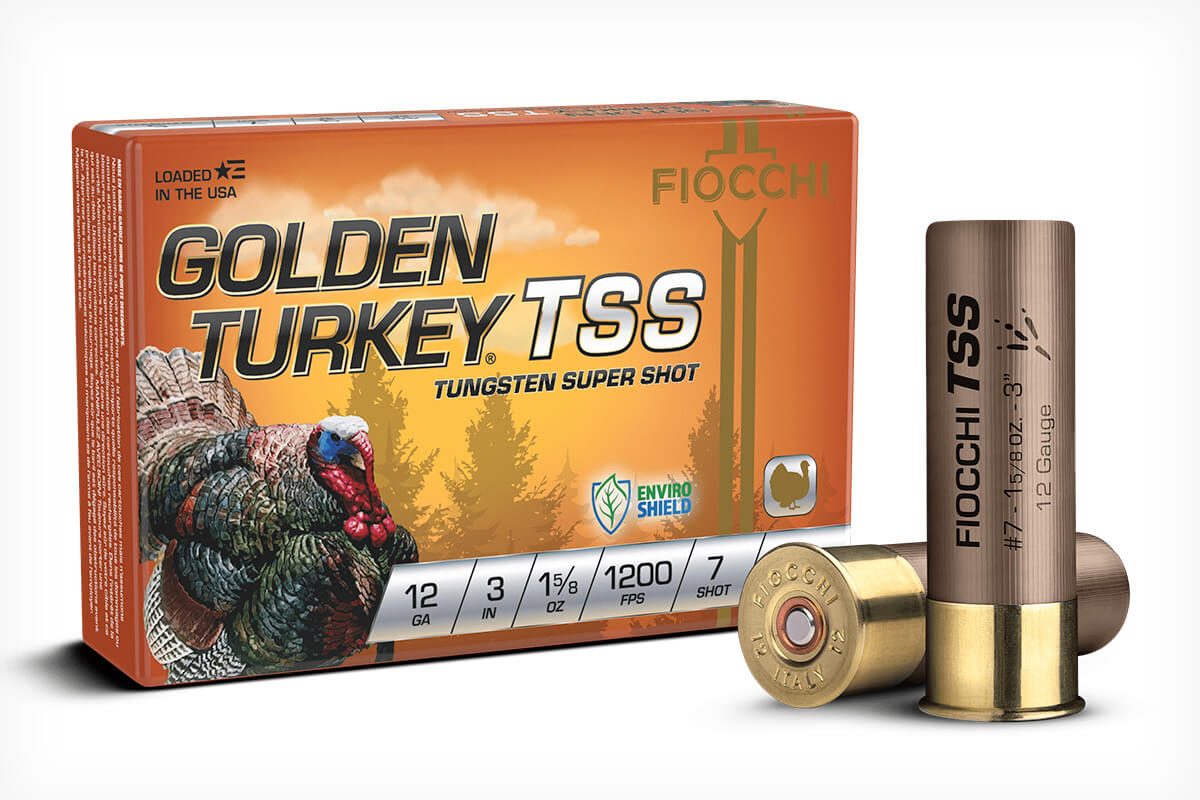
Detail Author:
- Name : Arvid Kirlin
- Username : alek13
- Email : cronin.brionna@jast.com
- Birthdate : 1995-12-11
- Address : 92952 O'Kon Loaf Apt. 441 East Samantha, SD 57805
- Phone : 954.502.9650
- Company : Von and Sons
- Job : Washing Equipment Operator
- Bio : Non nemo molestiae pariatur modi. Similique id culpa et harum. Ratione sed amet saepe velit rem dolor.
Socials
twitter:
- url : https://twitter.com/emayer
- username : emayer
- bio : Cupiditate voluptas quidem ut in maxime et molestiae porro. Et blanditiis iste qui voluptatibus quos. Iusto omnis aut et minima nisi.
- followers : 4235
- following : 338
linkedin:
- url : https://linkedin.com/in/edgardo_mayer
- username : edgardo_mayer
- bio : Distinctio odit et qui molestiae itaque quasi.
- followers : 1989
- following : 311
instagram:
- url : https://instagram.com/emayer
- username : emayer
- bio : Officiis blanditiis tenetur similique qui minus autem. Corrupti dolores hic quae perferendis nam.
- followers : 5221
- following : 288